Health
Rice University Develops Groundbreaking Machine Learning Algorithm
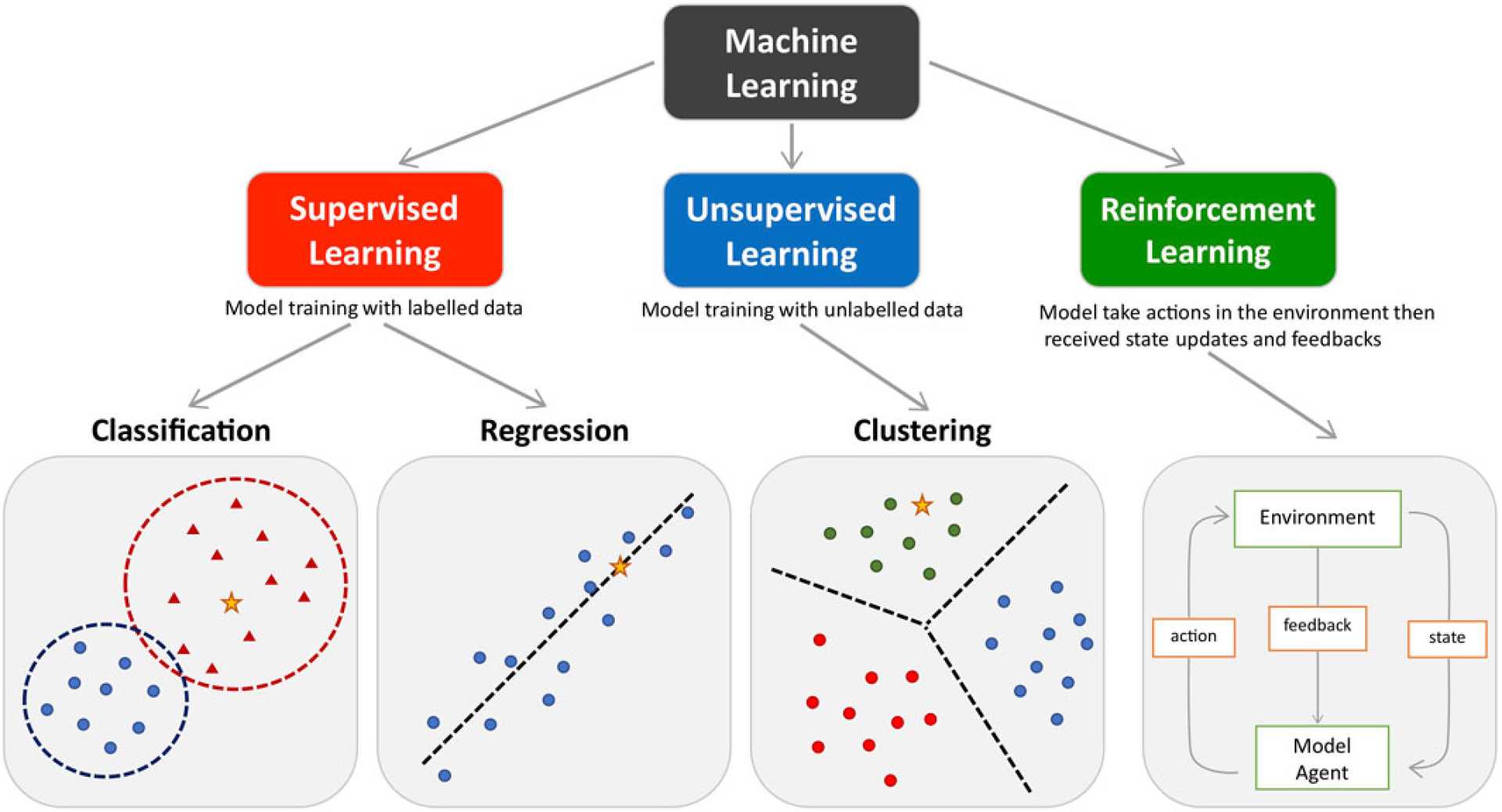
Houston, Texas – Researchers at Rice University have created an innovative machine learning algorithm that significantly enhances the interpretation of light signatures from molecules and disease biomarkers. This advancement could lead to quicker and more accurate medical diagnoses when detecting diseases, such as Alzheimer’s and COVID-19.
“Imagine being able to detect early signs of diseases just by shining a light on a drop of fluid or a tissue sample,” said Ziyang Wang, a doctoral student in electrical and computer engineering and the study’s first author. Their research is published in the journal ACS Nano.
Wang explained that each molecule scatters light in a unique way, creating an optical spectrum that serves as a fingerprint. Optical spectroscopy, a method that uses lasers to analyze how light interacts with materials, is essential in fields like chemistry and medicine. Yet, analyzing this spectral data can be both challenging and time-consuming.
The new algorithm, named Peak-Sensitive Elastic-net Logistic Regression (PSE-LR), improves the analysis of light-based data by classifying samples effectively. “The optical spectra of a biological sample can reveal much about what’s happening inside the body,” Wang remarked. “Faster, more accurate disease detection can lead to better treatments and save lives.”
PSE-LR not only classifies samples accurately but also provides transparency in its decision-making, which is often a limitation in other advanced machine learning models. The algorithm generates a “feature importance map” that identifies which aspects of the spectrum influenced classification outcomes. Wang likened PSE-LR to “a detective learning to find clues hidden in light signals.”
In tests, PSE-LR outperformed other models, especially in recognizing subtle spectral elements. “Most models either miss tiny details or are overly complex,” Wang stated. “We aimed for something that is both intelligent and understandable.”
The algorithm has demonstrated effectiveness in various real-world applications, including detecting minimal concentrations of the SARS-CoV-2 spike protein, classifying Alzheimer’s disease samples, and examining neuroprotective solutions in mouse brain tissue.
“Our tool can detect subtle signals that conventional methods often overlook,” said the study’s corresponding author, an associate professor at Rice. This innovation could lead to new diagnostics, biosensors, or nanodevices in the future.
<p“These findings may transform medical diagnostics and materials science, leading us towards a future where smart technologies help identify and address health issues quickly and efficiently,” Wang concluded.