News
John J. Hopfield and Geoffrey E. Hinton Honored for Pioneering Machine Learning Advances
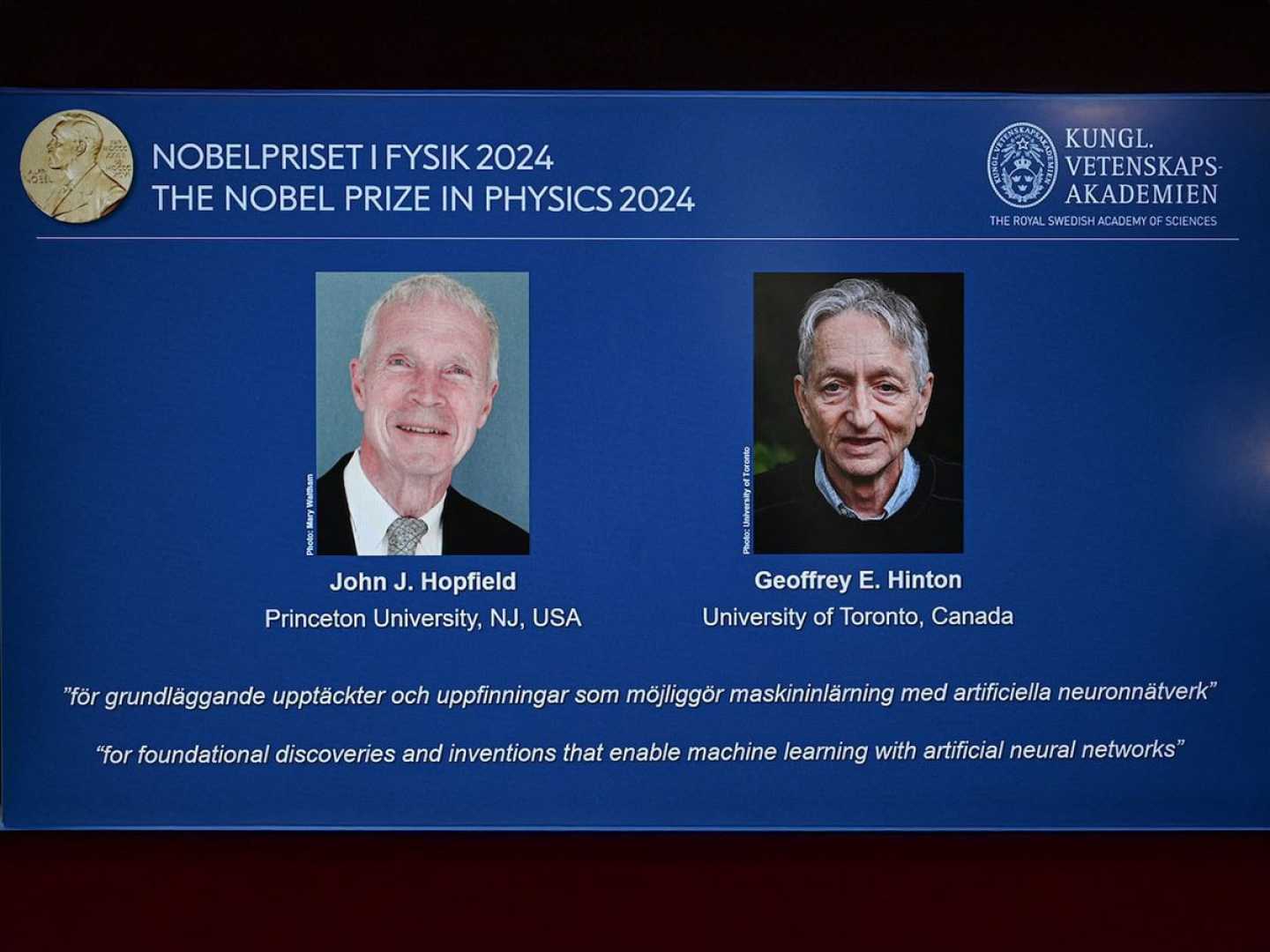
The Royal Swedish Academy of Sciences has announced that John J. Hopfield and Geoffrey E. Hinton are the recipients of this year’s Nobel Prize in Physics. The laureates have been recognized for their foundational work in developing techniques that have significantly contributed to machine learning, particularly the use of artificial neural networks.
John Hopfield’s contributions involve the creation of a network structure capable of storing and reconstructing information—methods reminiscent of associative memory used in the human brain. Geoffrey Hinton is honored for his pioneering method that allows computers to discover properties within data independently, a cornerstone of today’s large artificial neural networks.
Artificial neural networks, integral to modern machine learning and artificial intelligence, mimic cognitive processes such as memory and learning. Although machines lack the capacity for thought, they now simulate essential cognitive functions. The work of Hopfield and Hinton, grounded in physics, has been pivotal in advancing these technologies.
Hopfield developed his associative memory network in 1982, illustrating how such structures can retrieve stored patterns even from incomplete or altered data. His work, initially inspired by concepts from physics, established a method where interconnected nodes could store values and adjust connections to reproduce specific patterns.
Meanwhile, Hinton’s contributions expanded on these ideas by utilizing statistical physics. In 1985, he introduced the Boltzmann machine, which employs hidden layers of nodes to process input data. This model learns from examples, allowing it to identify and predict characteristics in new data sets.
The importance of their work has only been magnified with time. Since the resurgence of interest in artificial neural networks in the past two decades, their foundational ideas have facilitated the rise of deep learning. This has been instrumental in applications ranging from language translation to image recognition.
Hopfield began his neural network work after leaving Princeton University for the California Institute of Technology, leveraging advances in computing resources to develop his theories. Hinton, based at the University of Toronto, has seen his models evolve, contributing to widespread practical applications such as recommender systems and image recognition.
The acknowledgment of Hopfield and Hinton’s work by the Nobel Committee highlights the transformation of machine learning into a core technology with profound impacts across various fields, including the life sciences and engineering.