Health
New Algorithm Developed to Predict Seasonal Flu Virus Evolution
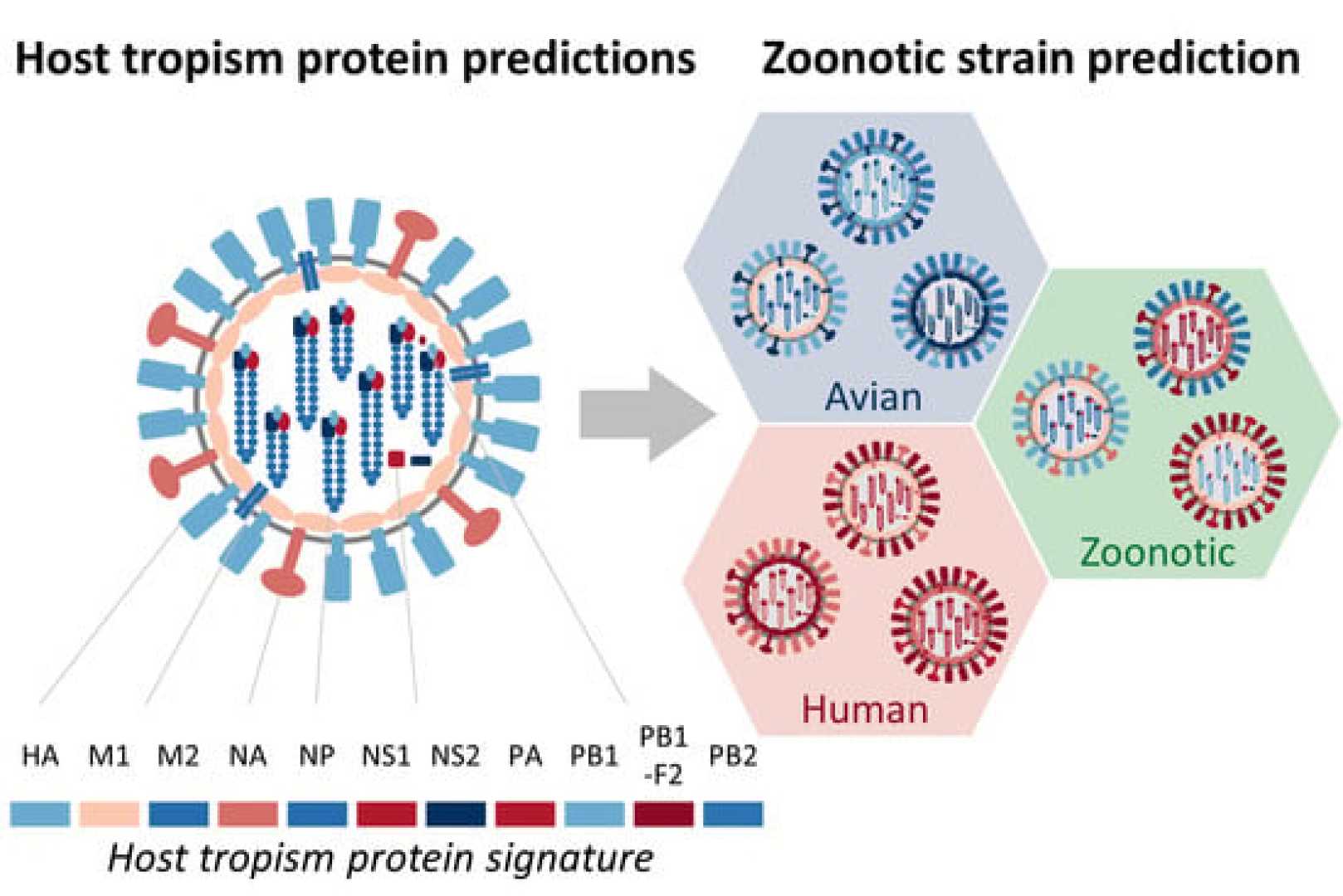
A team of scientists at the University of Georgia‘s Odum School of Ecology has created an innovative algorithm designed to predict how seasonal flu viruses are expected to evolve. This breakthrough may significantly enhance the speed at which seasonal flu vaccines can be updated, ultimately reducing the number of infections and fatalities attributed to the virus.
The findings, published in the journal Proceedings of the Royal Society B: Biological Sciences, introduce a tool known as the Novel Influenza Virus A Detector (NIAViD). This tool is capable of predicting changes in the seasonal flu virus with nearly 73% accuracy. The research was led by postdoctoral research associate Alpha Forna, who developed NIAViD to analyze flu virus sequence data to determine how emerging viruses might evade existing immunity within the population.
Annually, seasonal flu viruses infect approximately 1 billion individuals worldwide, contributing to an estimated 500,000 deaths, primarily among young children in developing nations. In the United States, millions are infected each year, with thousands succumbing to the illness. The economic burden of seasonal flu infections is projected to reach around $90 billion in the U.S. alone.
One major challenge in preventing seasonal flu infections is accurately forecasting how the virus will change, as explained by Forna. “Each flu virus, which we call influenza, has a hemagglutinin protein that initiates an infection when it binds to cells,” he stated. During the course of an infection, influenza viruses replicate, leading to small genetic alterations in the hemagglutinin protein, which are known as antigenic drift.
“Some of these changes may render an influenza virus unrecognizable to our immune system. This indicates that even if an individual has previously been infected with influenza or received a vaccine, they could still get infected by a virus that has a slightly altered hemagglutinin protein,” Forna added.
The use of machine learning—a method where computers learn autonomously—has become increasingly significant in infectious disease modeling. Over the course of two years, Forna built the NIAViD system, utilizing extensive datasets and training models to ensure accurate predictions. The algorithms developed specifically focus on a region of the hemagglutinin protein’s gene and several associated properties that measure various traits, such as a virus’s electrostatic charge.
Upon training the model on a selected data subset, its accuracy rate reached nearly 73%, comparable to or surpassing the performance of existing models. By concentrating on key attributes, NIAViD effectively identifies antigenic changes, consequently aiding in the timely updating of flu vaccines.
NIAViD was specifically designed based on an influenza virus that emerged during the 1968 pandemic originating in Hong Kong. Following the virus’s circulation through the global human population, laboratories began examining collected viruses to understand their responses to known protective antibodies. As viruses exhibiting immunity to either antibodies or vaccines were identified, it led to the discovery of clustered groups and the advancement of predictive modeling, guiding vaccine manufacturers toward emerging influenza lineages.
Co-author Justin Bahl, a professor at UGA’s College of Veterinary Medicine, emphasized the importance of previous research projects in vaccine development. He stated, “Clustering influenza viruses into specific groups to assess their immunity levels to vaccines was groundbreaking.”
However, Bahl pointed out that this research relied on a virus surveillance methodology that was labor-intensive, time-consuming, and sometimes unreliable. He expressed confidence that a more efficient and accurate method for predicting emerging seasonal flu variants was being realized through the application of an infectious disease machine-learning algorithm.
According to John Drake, Regents’ Professor and director of the Center for the Ecology of Infectious Diseases, the successful implementation of NIAViD opens new pathways for further research. “Its ability to rapidly identify antigenic variants can support the ongoing development of flu vaccines, enhancing public health readiness and response to seasonal flu,” Drake noted.
He also suggested that integrating NIAViD into surveillance systems could enable manufacturers to stay ahead of viral evolution, ensuring that vaccines effectively target the most current strains.