Tech
Apache Hive 4.0 Release Marks a Milestone in Data Management Technology
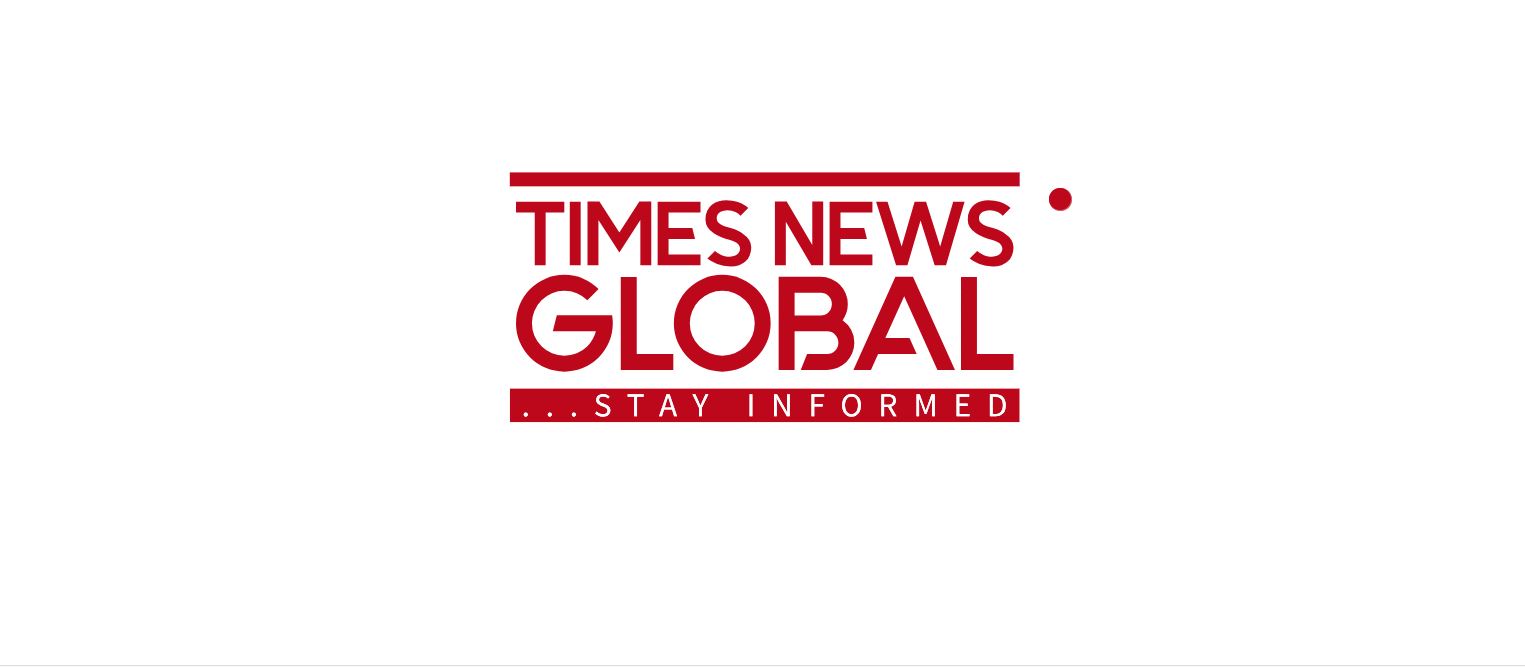
The Apache Software Foundation (ASF) has unveiled Apache Hive 4.0, a significant advancement in data lake and data warehouse technologies, further solidifying its position as a leading tool for big data processing. Since its establishment in 2010, Apache Hive has revolutionized data analytics and processing for organizations worldwide with its SQL-like query language.
The latest release, Hive 4.0, brings a host of improvements including seamless integration with Hive Iceberg tables, enhancing query performance, data integration, and scalability. This integration encompasses features like Branches and Tags support, Advanced Snapshot management, and Partition-level operations support.
Among the notable enhancements in Hive 4.0 are compaction mechanisms that streamline query performance and storage optimization for both Hive ACID and Iceberg tables. ACID, which ensures transaction integrity and reliability, receives upgraded transaction and locking capabilities in this version.
Additionally, users can now access official Apache Hive Docker images for simplified deployment and configuration, enhancing the management of Hive instances through Docker containers, a commendable initiative by ASF.
The Granite community has rolled out compiler enhancements in Hive 4.0, introducing support for HPL/SQL, scheduled queries, anti-joint support, and column histogram stats to elevate resource utilization and efficiency. The release also boasts new cost-based optimization (CBO) rules for performance optimization.
Other notable features include materialized views for expedited query processing, Apache Ozone support, enhanced replication capabilities for improved data distribution and disaster recovery, and runtime optimizations in Apache Tez and Apache Hive LLAP for accelerated data processing.
Ayush Saxena, an ASF Member and prominent Hive contributor, hails Hive 4.0 as a groundbreaking release, unlocking unparalleled capabilities for data management professionals seeking to handle massive datasets efficiently.